Empowering 50% Faster Drug Discovery with Automated Protein Analysis for a Leading Biotech
About the Client
This US-based biotech company is singularly focused on using innovations in gene therapy to lead the fight against devastating rare and prevalent diseases. They aim to find solutions to the limitations of conventional AAV capsids, promoters, and manufacturing approaches to improve tissue targeting, cell expression, packaging efficiency, and manufacturing quality, yields, and scalability.
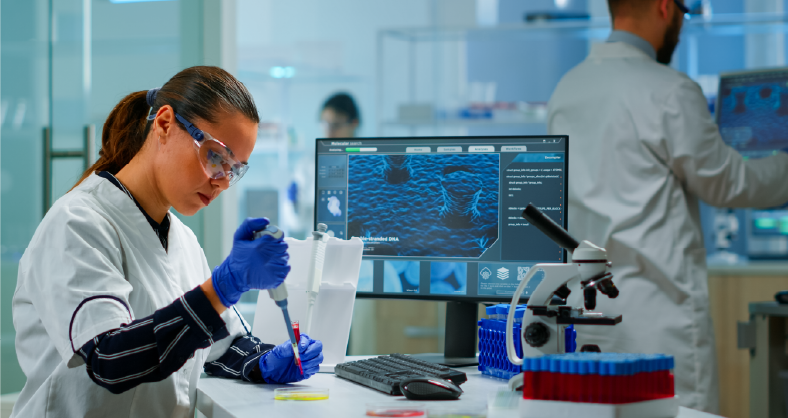
Their Challenges
The client relied on AlphaFold2, a machine learning model, to predict protein-protein interactions crucial for drug development. However, this caused:
Manual Process Limitations
They used the colabfold method for AlphaFold2, which hindered scalability for large datasets. Batch processing was needed to analyze datasets containing numerous protein sequences.
Time-Intensive Analysis
Analyzing protein structures was a time-consuming manual process, slowing down drug discovery progress.
The Solution
Automated Workflow Implementation
Searce built an automated solution on Google Cloud using Vertex AI Workbench for centralized model building and deployment. Custom Docker containers encapsulated AlphaFold2, enabling parallel processing of protein sequences.
IMPACT
Improved Efficiency & Scalability
Batch processing with Vertex AI Pipelines efficiently tackled large datasets (20×50 sequences), significantly reducing manual effort
IMPACT
Faster Drug Discovery
With a 50% reduction in protein prediction times, the client can now make faster, data-driven decisions, accelerating their drug development timeline
Orchestrated Inference and Secure Storage
Vertex AI Pipelines with Kubeflow Pipelines orchestrated the inference steps, ensuring smooth and efficient processing. Cloud Storage Buckets securely stored data and analysis results, providing easy access and management.
Success Story Highlights
The biotech’s drug discovery process achieved significant improvements with Searce’s AI solution. Protein prediction times were halved, thanks to multi-GPU inference and parallel execution on V100 GPUs. Monomer analysis time was reduced from 2 hours to 1 hour, while multimer sequence processing dropped from up to 9 hours to 8 hours. This translates to faster development and potentially lower costs for the client.
more case studies

Driving Logistics Innovation with Searce & Google Cloud

Yaantra partners with Searce & Google Cloud to help consumers with a single window stopover for smart gadgets in India
